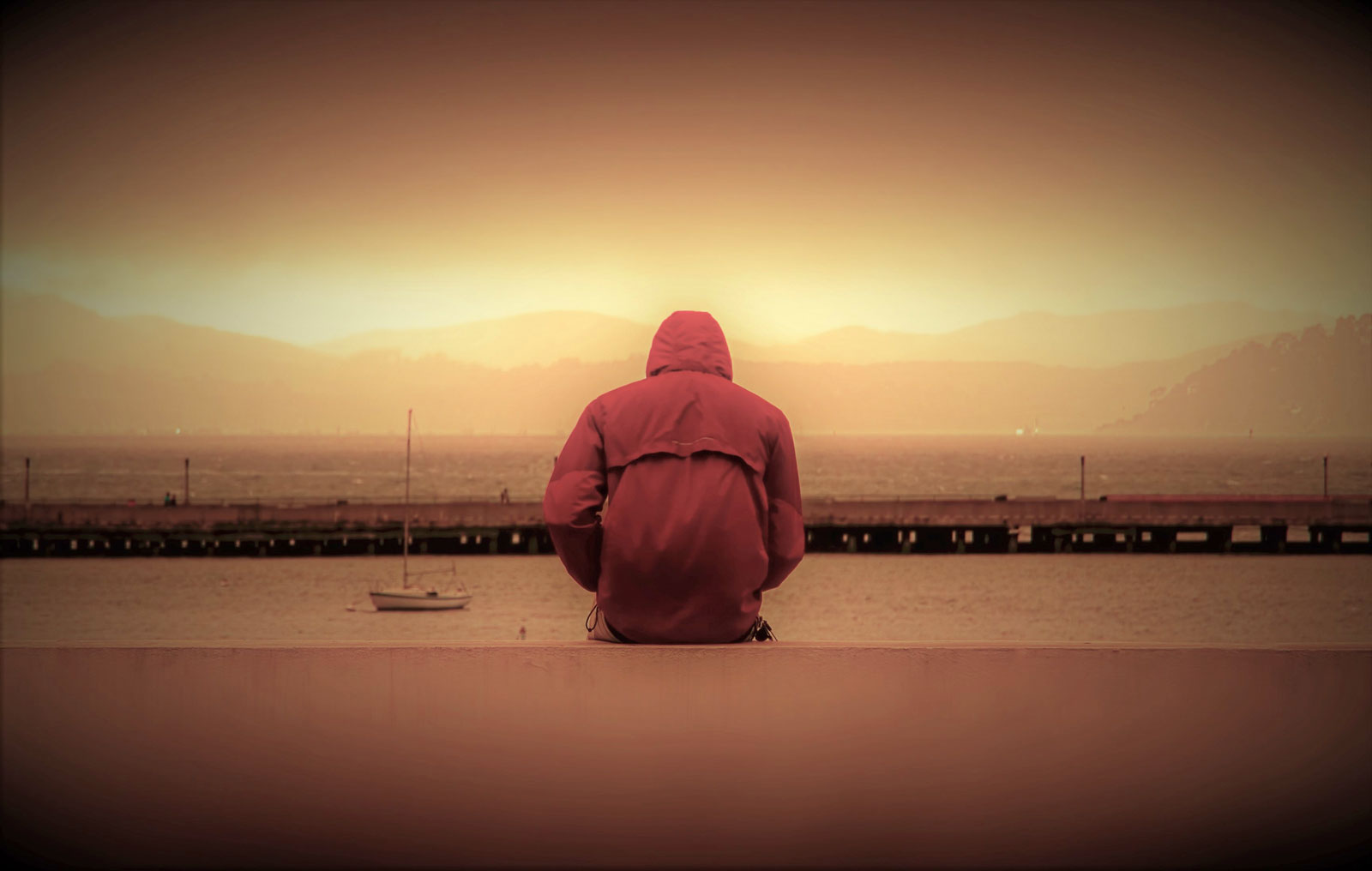 When it comes to identifying depression, doctors will traditionally ask patients specific questions about mood, mental illness, lifestyle and personal history, and use these answers to make a diagnosis. Now, researchers at MIT have created a model that can detect depression in people without needing responses to these specific questions, based instead on their natural conversational and writing style. According to the project's lead researcher, Tuka Alhanai, "The first hints we have that a person is happy, excited, sad, or has some serious cognitive condition, such as depression, is through their speech. If you want to deploy [depression-detection] models in scalable way ... you want to minimize the amount of constraints you have on the data you're using. You want to deploy it in any regular conversation and have the model pick up, from the natural interaction, the state of the individual." The researchers call the model "context-free", because there are no constraints in the types of questions that can be asked, or the type of responses that will be looked for. Using a technique called sequence modelling, the researchers fed the model text and audio from conversations with both depressed and non-depressed individuals. As the sequences accumulated, patterns emerged, such as the natural use of words such as "sad" or "down", and audio signals that are flatter and more monotone. "The model sees sequences of words or speaking style, and determines that these patterns are more likely to be seen in people who are depressed or not depressed," Alhanai says. "Then, if it sees the same sequences in new subjects, it can predict if they're depressed too." In tests, the model demonstrated a 77 percent success rate in identifying depression, outperforming nearly all other models – most of which rely on heavily structured questions and answers. The team says the model is intended to be a helpful tool to clinicians, since every patient speaks differently. "If the model sees changes maybe it will be a flag to the doctors," says co-researcher James Glass. In the future, the model could also power mobile apps that monitor a user's text and voice for mental distress and send alerts. This could be especially useful for those who can't get to a clinician for an initial diagnosis, due to distance, cost, or a lack of awareness that something may be wrong. The researchers also aim to test these methods on additional data from many more subjects with other cognitive conditions, such as dementia. "It's not so much detecting depression, but it's a similar concept of evaluating, from an everyday signal in speech, if someone has cognitive impairment or not," Alhanai says. Via: MIT Source: Detecting Depression with Audio/Text Sequence Modeling of Interviews [pdf]] via Engadget RSS Feed https://ift.tt/2PpmUiw |
Comments
Post a Comment